Popular information
Popular science background:
They have revealed proteins’ secrets through computing and artificial intelligence (pdf)
Populärvetenskaplig information:
De har avslöjat proteinernas hemligheter med hjälp av datorer och artificiell intelligens (pdf)
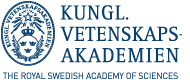
The Nobel Prize in Chemistry 2024
Chemists have long dreamed of fully understanding and mastering the chemical tools of life – proteins. This dream is now within reach. Demis Hassabis and John Jumper have successfully utilised artificial intelligence to predict the structure of almost all known proteins. David Baker has learned how to master life’s building blocks and create entirely new proteins. The potential of their discoveries is enormous.
They have revealed proteins’ secrets through computing and artificial intelligence
How is the exuberant chemistry of life possible? The answer to this question is the existence of proteins, which can be described as brilliant chemical tools. They are generally built from 20 amino acids that can be combined in endless ways. Using the information stored in DNA as a blueprint, the amino acids are linked together in our cells to form long strings.
Then the magic of proteins happens: the string of amino acids twists and folds into a distinct – sometimes unique – three-dimensional structure (Figure 1). This structure is what gives proteins their function. Some become chemical building blocks that can create muscles, horns or feathers, while others may become hormones or antibodies. Many of them form enzymes, which drive life’s chemical reactions with astounding precision. The proteins that sit on the surfaces of cells are also important, and function as communication channels between the cell and its surroundings.

It is hardly possible to overstate the potential encompassed by life’s chemical building blocks, these 20 amino acids. The Nobel Prize in Chemistry 2024 is about understanding and mastering them at an entirely new level. One half of the prize goes to Demis Hassabis and John Jumper, who have utilised artificial intelligence to successfully solve a problem that chemists wrestled with for over 50 years: predicting the three-dimensional structure of a protein from a sequence of amino acids. This has allowed them to predict the structure of almost all 200 million known proteins. The other half of the prize is awarded to David Baker. He has developed computerised methods for achieving what many people believed was impossible: creating proteins that did not previously exist and which, in many cases, have entirely new functions.
The Nobel Prize in Chemistry 2024 recognises two different discoveries but, as you will see, they are closely linked. To understand the challenges this year’s laureates have overcome, we must look back to the dawn of modern biochemistry.
The first grainy pictures of proteins
Chemists have known since the nineteenth century that proteins are important for life’s processes, but it took until the 1950s for chemical tools to be precise enough for researchers to start exploring proteins in more detail. Cambridge researchers John Kendrew and Max Perutz made a groundbreaking discovery when, at the end of the decade, they successfully used a method called X-ray crystallography to present the first three-dimensional models of proteins. In recognition of this discovery, they were awarded the Nobel Prize in Chemistry in 1962.

Subsequently, researchers have primarily used X-ray crystallography – and often a great deal of effort – to successfully produce images of around 200,000 different proteins, which laid the foundation for the Nobel Prize in Chemistry 2024.
A riddle: how does a protein find its unique structure?
Christian Anfinsen, an American scientist, made another early discovery. Using various chemical tricks, he managed to make an existing protein unfold and then fold itself up again. The interesting observation was that the protein assumed exactly the same shape every time. In 1961, he concluded that a protein’s three-dimensional structure is entirely governed by the sequence of amino acids in the protein. This led to him being awarded the Nobel Prize in Chemistry in 1972.
However, Anfinsen’s logic contains a paradox, which another American, Cyrus Levinthal, pointed out in 1969. He calculated that even if a protein only consists of 100 amino acids, in theory the protein can assume at least 1047 different three-dimensional structures. If the chain of amino acids were to fold randomly, it would take longer than the age of the universe to find the correct protein structure. In a cell, it just takes a few milliseconds. So how does the string of amino acids actually fold?
Anfinsen’s discovery and Levinthal’s paradox implied that folding is a predetermined process. And – importantly – all the information about how the protein folds must be present in the amino acid sequence.
Throwing down the gauntlet for the great challenge of biochemistry
The above insights led to another decisive realisation – if chemists know a protein’s amino acid sequence, they should be able to predict the protein’s three-dimensional structure. This was an exciting idea. If they succeeded, they would no longer have to use fiddly X-ray crystallography and could save masses of time. They would also be able to generate structures for all the proteins where X-ray crystallography was not applicable.
These logical conclusions threw down the gauntlet for what has become the great challenge of biochemistry: the prediction problem. To encourage more rapid development in the field, in 1994 researchers started a project called Critical Assessment of Protein Structure Prediction (CASP), which developed into a competition. Every other year, researchers from around the globe were given access to sequences of amino acids in proteins whose structures had just been determined. However, the structures were kept secret from the participants. The challenge was to predict the protein structures based on the known amino acids sequences.
CASP attracted many researchers, but solving the prediction problem proved incredibly difficult. The correspondence between the predictions researchers entered in the competition and the actual structures hardly improved at all. The breakthrough only occurred in 2018, when a chess master, neuroscience expert and pioneer in artificial intelligence entered the field.
Boardgame master enters the Protein Olympics
Let’s take a quick look at Demis Hassabis’ background: he started playing chess at the age of four and achieved master level as a 13-year-old. In his teens, he started a career as a programmer and successful games developer. He began exploring artificial intelligence and took on neuroscience, where he made several revolutionary discoveries. He used what he learned about the brain to develop better neural networks for AI. In 2010 he co-founded DeepMind, a company that developed masterful AI models for popular boardgames. The company was sold to Google in 2014 and, two years later, DeepMind came to global attention when the company achieved what many then believed to be the holy grail of AI: beating the champion player of one of the world’s oldest boardgames, Go.
However, for Hassabis, Go was not the goal, it was the means for developing better AI models. After this victory, his team were ready to tackle problems of greater importance for humanity, so in 2018 he registered for the thirteenth CASP competition.
An unexpected win for Demis Hassabis’ AI model
In previous years, the protein structures that researchers predicted for CASP had achieved an accuracy of 40 per cent, at best. With their AI model, AlphaFold, Hassabis’ team reached almost 60 per cent. They won, and the excellent result took many people by surprise – it was unexpected progress, but the solution was still not good enough. For success, the prediction had to have an accuracy of 90 per cent when compared to the target structure.

Hassabis and his team continued developing AlphaFold – but, however hard they tried, the algorithm never quite went all the way. The hard truth was that they had come to a dead end. The team was tired, but one relatively new employee had decisive ideas about how the AI model could be improved: John Jumper.
John Jumper picks up the gauntlet of biochemistry’s big challenge
John Jumper’s fascination with the universe was what made him start studying physics and mathematics. However, in 2008, when he started working at a company that used supercomputers to simulate proteins and their dynamics, he realised that knowledge of physics could help solve medical problems.
Jumper took this newly acquired interest in proteins with him when, in 2011, he began his doctorate in theoretical physics. To save computer capacity – something that was in short supply at the university – he started developing simpler and more ingenious methods for simulating protein dynamics. Soon, he too picked up the gauntlet of biochemistry’s big challenge. In 2017, he had recently completed his doctorate when he heard rumours that Google DeepMind had, in great secrecy, started to predict protein structures. He sent them a job application. His experience of protein simulation meant he had creative ideas about how to improve AlphaFold so, after the team had started to tread water, he was promoted. Jumper and Hassabis co-led the work that fundamentally reformed the AI model.
Astounding results with a reformed AI model
The new version – AlphaFold2 – was coloured by Jumper’s knowledge of proteins. The team also started to use the innovation behind the recent enormous breakthrough in AI: neural networks called transformers. These can find patterns in enormous amounts of data in a more flexible manner than previously, and efficiently determine what should be focused on to achieve a particular goal.
The team trained AlphaFold2 on the vast information in the databases of all known protein structures and amino acid sequences (Figure 2) and the new AI architecture started delivering good results in time for the fourteenth CASP competition.
In 2020, when CASP’s organisers evaluated the results, they understood that biochemistry’s 50-year-old challenge was over. In most cases, AlphaFold2 performed almost as well as X-ray crystallography, which was astounding. When one of CASP’s founders, John Moult, concluded the competition on 4 December 2020, he asked – what now?
We will return to that. Now we are going to go back in time and shine a light on another participant in CASP. Let’s present the other half of the Nobel Prize in Chemistry 2024, which deals with the art of creating new proteins from scratch.
A textbook about the cell makes David Baker change direction
When David Baker started studying at Harvard University, he chose philosophy and social science. However, during a course in evolutionary biology he came across the first edition of the now classic textbook Molecular Biology of the Cell. This led to him changing his direction in life. He began to explore cell biology and eventually he became fascinated by protein structures. When, in 1993, he started as group leader at the University of Washington in Seattle, he took on biochemistry’s great challenge. Using clever experiments, he began to explore how proteins fold. This provided insights he took with him when, at the end of the 1990s, he began to develop computer software that could predict protein structures: Rosetta.
Baker made his debut in the CASP competition in 1998 using Rosetta and, in comparison to other participants, it did really well. This success led to a new idea – that David Baker’s team could use the software in reverse. Instead of entering amino acid sequences in Rosetta and getting protein structures out, they should be able to enter a desired protein structure and obtain suggestions for its amino acid sequence, which would allow them to create entirely new proteins.
Baker becomes a protein constructor
The field of protein design – where researchers create bespoke proteins with new functions – began to take off at the end of the 1990s. In many cases, researchers tweaked existing proteins, so they could do things like breaking down hazardous substances or functioning as tools in the chemical manufacturing industry.
However, the range of natural proteins is limited. To increase the potential for obtaining proteins with entirely new functions, Baker’s research group wanted to create them from scratch. As Baker said, “If you want to build an airplane, you don’t start by modifying a bird; instead, you understand the first principles of aerodynamics and build flying machines from those principles.”

A unique protein sees the light of day
The field in which entirely new proteins are constructed is called de novo design. The research group drew a protein with an entirely new structure, and then had Rosetta compute which type of amino acid sequence could result in the desired protein. To do this, Rosetta searched a database of all known protein structures, and looked for short fragments of proteins that had similarities with the desired structure. Using fundamental knowledge of proteins’ energy landscape, Rosetta then optimised these fragments and proposed an amino acid sequence.
To investigate how successful the software was, Baker’s research group introduced the gene for the proposed amino acid sequence in bacteria that produced the desired protein. Then they determined the protein structure using X-ray crystallography.
It turned out that Rosetta really could construct proteins. The protein that the researchers developed, Top7, had almost exactly the structure they had designed.
Spectacular creations from Baker’s laboratory
Top7 was a bolt from the blue for the researchers working on protein design. Those who had previously created de novo proteins had only been able to imitate existing structures. Top7’s unique structure did not exist in nature. Also, with its 93 amino acids, the protein was larger than anything previously produced using de novo design.
Baker published his discovery in 2003. This was the first step in something that can only be described as an extraordinary development; a few of the many spectacular proteins created in Baker’s laboratory can be seen in Figure 4. He also released the code for Rosetta, so a global research community has continued to develop the software, finding new areas of application.
It is time to tie up the loose ends of the Nobel Prize in Chemistry 2024. What now?
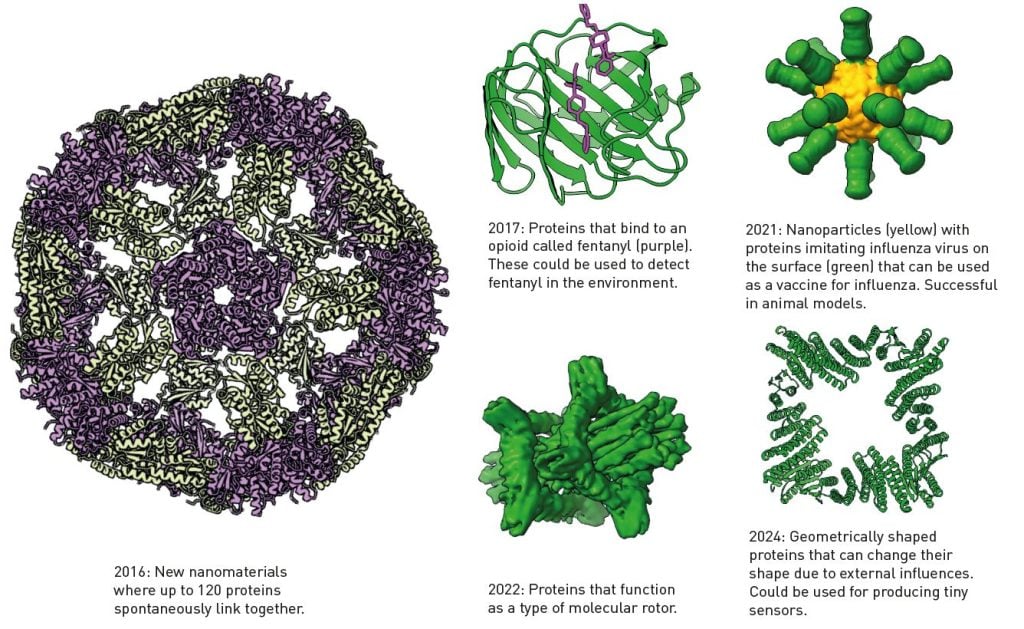
Work that once took years now takes just a few minutes
When Demis Hassabis and John Jumper had confirmed that AlphaFold2 really worked, they calculated the structure of all human proteins. Then they predicted the structure of virtually all the 200 million proteins that researchers have so far discovered when mapping Earth’s organisms.
Google DeepMind has also made the code for AlphaFold2 publicly available, and anyone can access it. The AI model has become a gold mine for researchers. By October 2024, AlphaFold2 had been used by more than two million people from 190 countries. Previously, it often took years to obtain a protein structure, if at all. Now it can be done in a few minutes. The AI model is not perfect, but it estimates the correctness of the structure it has produced, so researchers know how reliable the prediction is. Figure 5 shows a few of the many examples of how AlphaFold2 helps researchers.
After the 2020 CASP competition, when David Baker realised the potential of transformer-based AI models, he added one to Rosetta, which has also facilitated the de novo design of proteins. In recent years, one incredible protein creation after the other has emerged from Baker’s laboratory (Figure 4).

Dizzying development for the benefit of humankind
Proteins’ amazing versatility as chemical tools is reflected in the vast diversity of life. That we can now so easily visualise the structure of these small molecular machines is mind boggling; it allows us to better understand how life functions, including why some diseases develop, how antibiotic resistance occurs or why some microbes can decompose plastic.
The ability to create proteins that are loaded with new functions is just as astounding. This can lead to new nanomaterials, targeted pharmaceuticals, more rapid development of vaccines, minimal sensors and a greener chemical industry – to name just a few applications that are for the greatest benefit of humankind.
Further reading
Additional information on this year’s prizes, including a scientific background in English, is available on the website of the Royal Swedish Academy of Sciences, www.kva.se, and at www.nobelprize.org, where you can watch video from the press conferences, the Nobel Lectures and more. Information on exhibitions and activities related to the Nobel Prizes and the Prize in Economic Sciences is available at www.nobelprizemuseum.se.
The Royal Swedish Academy of Sciences has decided to award the Nobel Prize in Chemistry 2024
with one half to
DAVID BAKER
Born 1962 in Seattle, WA, USA. PhD 1989 from University of California, Berkeley, CA, USA. Professor at University of Washington, Seattle, WA, USA and Investigator, Howard Hughes Medical Institute, USA.
“for computational protein design”
and the other half jointly to
DEMIS HASSABIS
Born 1976 in London, UK. PhD 2009 from University College London, UK. CEO of Google DeepMind, London, UK.
JOHN JUMPER
Born 1985 in Little Rock, AR, USA. PhD 2017 from University of Chicago, IL, USA. Senior Research Scientist at Google DeepMind, London, UK.
“for protein structure prediction”
Science Editors: Peter Brzezinski, Heiner Linke, Johan Åqvist, the Nobel Committee for Chemistry
Text: Ann Fernholm
Translator: Clare Barnes
Illustrations: Johan Jarnestad, Terezia Kovalova
Editor: Vincent von Sydow
© The Royal Swedish Academy of Sciences
Nobel Prizes and laureates
Six prizes were awarded for achievements that have conferred the greatest benefit to humankind. The 12 laureates' work and discoveries range from proteins' structures and machine learning to fighting for a world free of nuclear weapons.
See them all presented here.